Learning Introspective Control (LINC) Military Systems to Adapt to the Unexpected
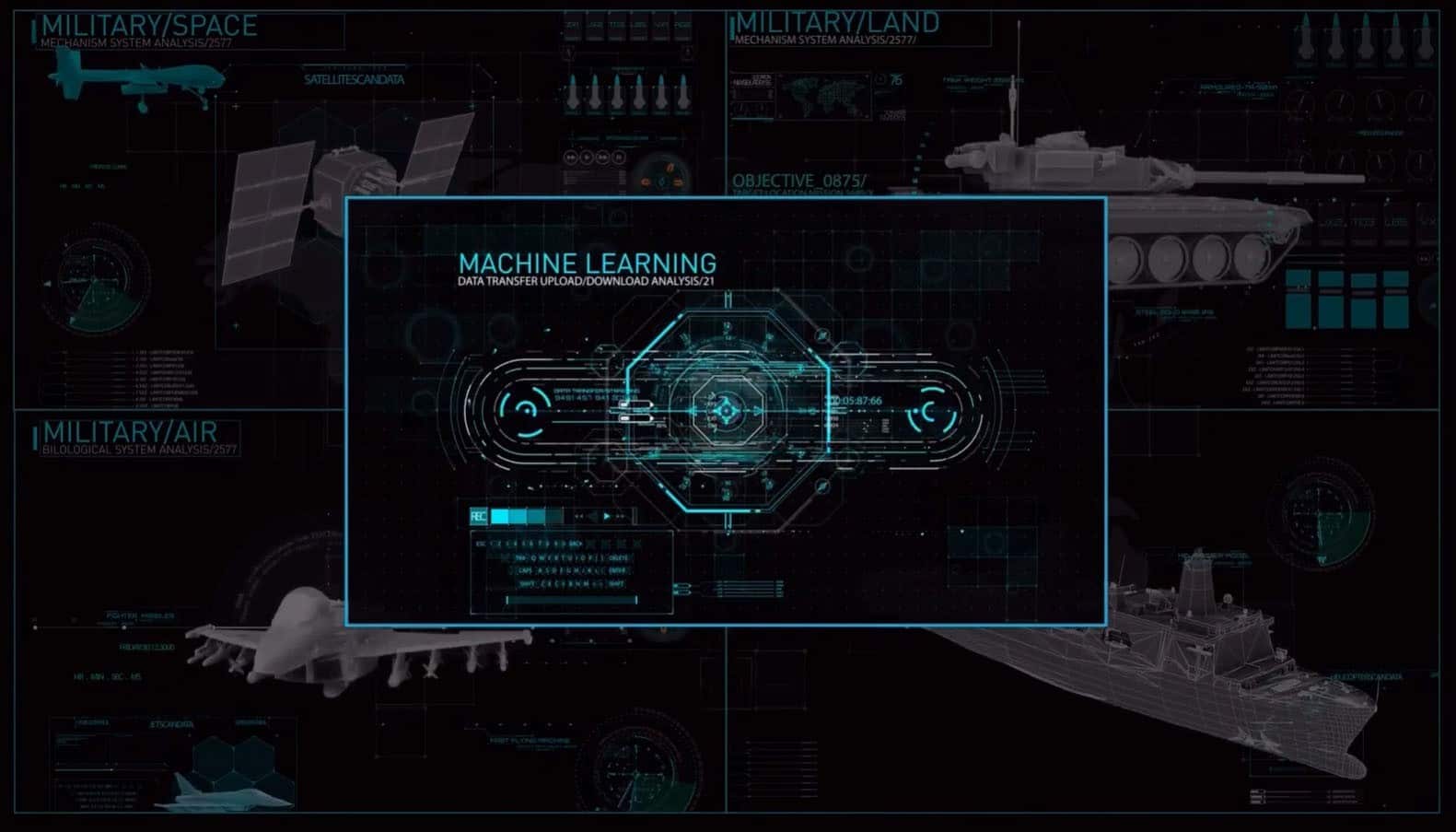
Many complex, cyber-physical military systems are designed to last for decades but their expected functionality and capabilities will likely evolve over time, prompting a need for modifications and adaptation.
High Mobility Multipurpose Wheeled Vehicles (HMMWV), for example, had a design life of 15 years, but are now undergoing modernization to extend the average age of the fleet to 37+ years. At design time, these systems are built to handle a range of expected operating environments and parameters.
Adapting them is currently done in an improvisational manner – often involving custom-tailored aftermarket remedies, which are not always commonly available, require a skilled technician to install, and can take months or even years to procure. Further, as they evolve and are placed outside of their original design envelop these systems can fail unexpectedly or become unintentionally dangerous.
“Today, we start with exquisitely built control systems but then someone needs to add something or make a modification – all of which results in changes to the safe operating limits,” said DARPA program manager John-Francis Mergen. “These changes are done in a way that wasn’t anticipated – or more likely couldn’t have been anticipated – by the original designers. Knowing that military systems will undoubtedly need to be altered, we need greater adaptability.”
In response, DARPA developed the Learning Introspective Control (LINC) program. The program aims to develop machine learning (ML)-based introspection technologies that enable systems to adapt their control laws as they encounter uncertainty or unexpected events. The program also seeks to develop technologies to communicate these changes to a human or AI operator while retaining operator confidence and ensuring continuity of operations.
“When a system ‘wakes up’ in a different space, it needs to be able to realize there are things it can’t do anymore or new things it can, and ‘learn’ how to adapt to its new operating reality,” noted Mergen. “With LINC, we want to provide physical systems with the ability to figure out what is still feasible, alert the operator, and then help them operate in that new space.”
Developing this military systems, LINC technologies, will require addressing a specific set of challenges related to learning control and communicating situational awareness to the operator. Current state of the art (SOTA) ML approaches are not robust to unknown or unstructured parameter uncertainty, owing largely to the bounds set on their operation at design time as well as their reliance on fixed assumptions about their operating model. Further, complex systems – like drone swarms – are unable to rapidly converge on a common solution. When damage occurs to a single drone, the swarm is unable to uniformly adapt, potentially resulting in a failed operator or unsafe operating conditions.
LINC’s first research area will seek to overcome existing limitations in learning models and ML techniques that currently hamper system adaptation. The program will explore how to provide a system with the ability to sense change and then reconstitute control using only onboard sensors and actuators. LINC aims to develop new control regimes that detect and characterize changes in the system’s operations in real-time, rapidly find solutions for reconstituting control under these changing conditions, and then calculate operating limits to identify a safe operating envelope.
“The idea is that you have a plethora of indigenous sensors on the system, and you can use these to determine and define a new set of control laws. With those new laws, you can then calibrate the system,” said Mergen.
Another challenge area LINC seeks to address is around operator communications. Today, operators are not often provided with sufficient explanations or guidance around a system’s behavior or it’s situation-specific operating limits. Existing cues to operators about system dynamics don’t always provide options, making it difficult for an operator to appropriately trust the information its receiving. Further, interpreting current system diagnostics displays, which are not always intuitive, creates additional cognitive load for human operators. This further erodes operator trust and can lead to misunderstanding, confusion, and incorrect actions.
A second research area will focus on improving how situational awareness and guidance are shared with the operator. This area will explore ways of translating and effectively communicating the operational information generated by the dynamic model developed under the first research area. The resulting technologies must be able to provide the operator – whether human or AI – with updates on the operating status of the system as well as cues for safe actions. Further, they must be able to help retain operator trust by providing optionality and explainability around what’s happening “under the hood.”
A third research area will focus on testing and evaluating the resulting technologies. LINC expects to use demonstration platforms that will evolve in sophistication and complexity throughout the life of the program – starting with a realistic physical model and progressing to a military-relevant system in the program’s final phase.
Interested proposers will have an opportunity to learn more about this military systems, the Learning Introspective Control (LINC) program during a Proposers Day, which will be held on August 26, 2021, from 9:00 AM to 2:00 PM (ET) both at the DARPA Conference Center, located at 675 N. Randolph Street, Arlington, Virginia, 22203, and virtually through Zoom. Advance registration is required to attend. To learn more, please visit: https://sam.gov/opp/69db6bff225344f481f229edc1e2b97a/view
The LINC Broad Agency Announcement is forthcoming and will be published on the System for Award Management (SAM) website at https://beta.sam.gov/.